The AI-based visual inspection helps in redefining quality control in manufacturing - Vinodh Venkatesan, Co-founder and COO, Jidoka Technologies
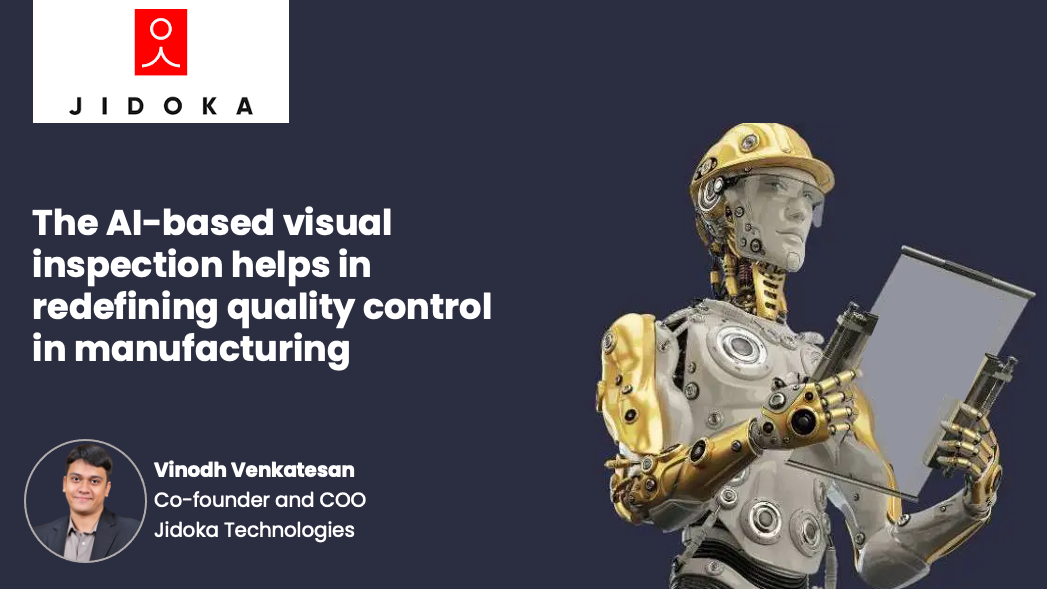
Cost
of Poor Quality (CoPQ) is certainly a key performance indicator of efficiency
in manufacturing. In cases where the
Quality Control (QC) processes are not monitored or controlled accurately, the
impact on the organization’s bottom line is quite significant. Inadequate quality checks can lead to huge
losses for manufacturers, running into millions of dollars, depending on the
scale of the operations. Manufacturing
quality can be driven higher by Artificial Intelligence (AI), with the
establishment of superior yields, lower scrap rates, positive customer
feedback, and ensuring receipt of good quality materials from suppliers. QC practices
have evolved from being completely manual to traditional machine vision with
the introduction of computers and automation and the AI-powered visual
inspection of today.
The
traditional machine vision automated systems are based on human-defined rules
that are established in a standard computing programming setting. Although they reduce human error, this system
is not able to detect complex defects in constantly changing environments.
Today, manufacturers who want to address these challenges need not rely so much on either QC inspectors or rigid rule-based traditional machine vision. They can now choose a better option of leveraging AI-powered visual inspection systems which include machine learning (ML) and deep learning (DL) technologies for powering the QC solution as part of the shift to Industry 4.0 or shopfloor digitization. These solutions can manage complex applications at a faster pace compared to the traditional machine vision system. With this new entrant, there is a reduction in the number of customer complaints, an increase in yield, a decrease in human fatigue and stress, and improved traceability while enhancing the brand’s reputation.
Advantages
of AI-based visual inspection
AI can help in achieving automated quality testing, effortlessly. Its advanced image recognition techniques for visual inspection and fault detection can significantly increase productivity. By using image recognition, AI-based visual inspection can help in increasing defect detection rates close to almost 100 percent consistently as compared to human inspection. Scrap rates and testing costs are reduced too, thereby enhancing the yield or throughput.
Overcoming
AI-model limitations
Along
with several benefits AI visual systems also present some challenges during
their deployment and while scaling. Manufacturers sometimes report a lack of data resources which is an
obstacle to the effective deployment of AI solutions. Models from a limited amount of data will not
support tools that are designed for large manufacturing plants, to function
reliably and consistently.
However,
by gathering the required number of samples, and adopting a clear and
disciplined process of data labeling, manufacturers can eliminate ambiguous
defect detections and labeling instructions in addition to inconsistencies exhibited
by the labeler, ensuring clean, non-noise data.
By having
data-centric AI models as opposed to model-centric ones, the defects are generalized
but the specifics for each organization are maintained independently. This helps
in reducing the deployment times and achieving the best accuracies.
In a shopfloor environment, if two or three Quality Control experts disagree on identifying the defect and coming up with a common definition, training AI from them for good performance is not possible. Manufacturers should rely on objective dashboards that can support human inspectors to come up with clear unambiguous defect definitions and categorize defects accurately. Many a time the changes that occur in environments, both external and internal can impact the performance of the AI-based visual inspection systems. Here, the inspection team has to label and retrain the model according to the evolving product requirements and consistently look out for abrupt changes.
Implementing
AI visual inspection systems
At the outset, the type of defects to be detected has to be clearly defined by asking a few key questions. These could include, the kind of defects the system should detect, the size of the object inspected, and the typical sizes of the defects, how it should notify the user about the defect, and whether the inspection has to be in real-time or not. The data science engineers should gather and prepare data that can be used to train AI. It should be cleaned and checked for anomalies and false signals while ensuring its relevance. A deep learning model can be established by either developing it from the scratch or using pre-trained models. Then the developed visual inspection model has to be trained and deployed. Furthermore, the model should be subjected to continuous improvement upon deployment by gathering new data and model re-training.
Enhanced
FMCG packaging with AI-enabled visual inspection
To
understand the benefits of AI-based visual inspection systems further, let us
consider the QC process at a milk packaging company. Here, there is a strong possibility of milk
packets in crates being placed randomly by the workers leading to inaccuracy in
counting and sorting. AI can be used to
recognize the Stock Keeping Unit (SKU) or grade of packets irrespective of the
loading orientation or positioning of milk packets. AI-based visual inspection solution can be
used by integrating a camera and software with the machine where inspection of
milk packets is done before the crates are preserved in cold storage. Compared
to conventional vision techniques, AI-backed algorithms can look at thousands
of data points visible on the crates with milk packets. This includes the size
of the packets, the color or design on them, the position and location of the
characters printed on them, etc., among others. This method closely mimics what
a trained human brain is subconsciously processing while assuming or judging
the SKU and/or grade of the milk packets. Computer vision is, therefore, able
to offer solutions in problem areas that were previously impossible through rule-based
machine vision programs. This process
can establish near 100 percent count accuracy in SKU grade and quantity
verification with 100 percent inspection without depending on human judgment thereby
eliminating errors that could occur due to the fatigue factor. With automated reporting, the need for manual
counting of crates in cold storage is eliminated. With this, the organization can easily
determine exactly how many crates of each grade and SKU are stored, which can
then be compared against produced quantity and dispatched quantity ensuring
accountability and traceability in the system. For example, the system can count at 1,200 crates per hour (an avg. 50
packets per SKU), sorting 15 different varieties.
Among
many other use-cases, here is another example where the AI-enabled QC model is
used for the inspection of biscuit packets, after primary packaging and before
secondary packaging into larger plastic bags, where several biscuit packets are
eventually placed. An AI solution used here
will include integrating one camera, parts ejection, and software with the
machine, resulting in zero error. This
process can replace the manual inspection done to switch the placements of wrongly
oriented packets which could otherwise lead to an increase in the error
rate. In the incorrectly placed packets,
the chances of biscuits getting broken inside the boxes and during transit are
high.
Additionally,
for the inspection before the packaging stage, computer vision could be used in
identifying cracks in the biscuits and ensuring the sandwiching process is done
right without any errors, such as wrong orientation. The computing power and
automation speed can be boosted so high that even speeds of 4000+ inferences
per minute are made possible.
These AI-based processes also help in achieving significant cost savings.
For improving the QC processes, manufacturers across FMCG and other industry verticals are increasingly leveraging AI-based systems that are more promising today. The manufacturing sector has greater potential for benefiting from the AI systems leading to a significant boost in quality control, performance, throughput, and business outcomes.