Data trends that defined 2022 – a review - Anjna Bhati, Director - Data Analytics & AI, BluePi Consulting
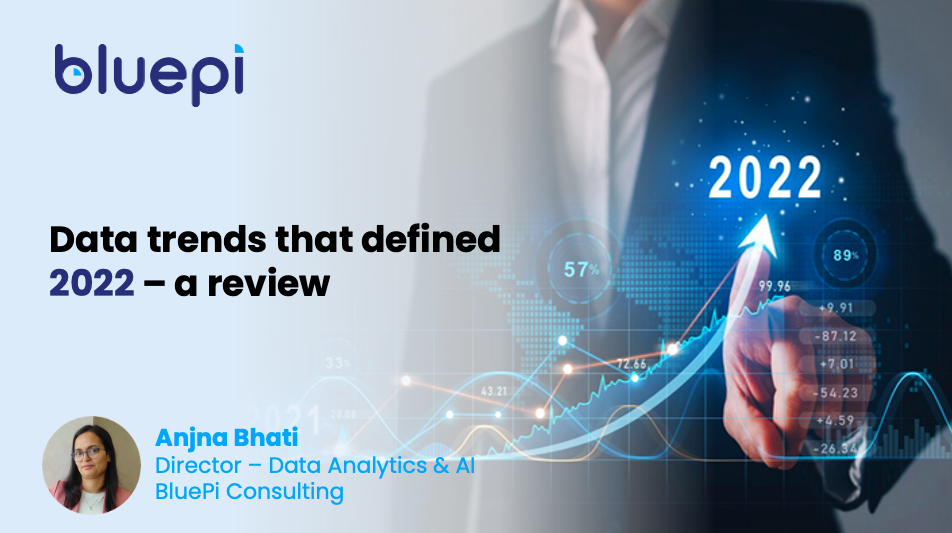
In the current digital world, new digital experiences
are sought after with technology enabling them constantly and ‘data’ driving
the whole exercise. Data science, Big
Data Analytics, and others are supporting decision-making and data-focused
product offerings. Businesses have to
rely on near real-time data to draw actionable insights for better outcomes. Data Analytics is today enabling large
enterprises, SMEs, and Startups to achieve operational excellence by optimizing
processes, enhancing customer experiences, and enabling the implementation of an
effective marketing strategy.
Some key developments in the data domain in the past
year are summarized in the next few lines.
Modernizing legacy applications was the top
priority
A significant number of organizations accelerated their digital transformation efforts to upgrade their business processes to deliver better customer experience, increase employee productivity and establish business reliability. For automating content ingestion, hyper-automation technologies like Natural Language Technology, Optical Character Recognition, and Conversational AI were in demand. These helped to reduce operational costs significantly. Composability, the system design principle, that deals with the interrelationship of components, was leveraged by many enterprises. It enabled high flexibility, agility, and improvement in time-to-market. Duplicacies of applications and data were reduced and there was a continuous improvement too with it. Business Process Automation solutions were used to automate multi-step business transactions and Cloud computing with open-source platforms accelerated the app modernization journey.
Data Lakes adoption for Big Data Storage
The unstoppable increase in data generation has led
to organizations opting for data lakes more than before to store structured and
unstructured data sets in their native format.
Data lake houses as primary storage of data became an economical option
with the increase in remote and hybrid working.
The growing demand for cloud-enabled data platforms, the need for
actionable business insights, and the higher speed for data retrieval drive the
data lakes market. Furthermore, data manipulation is efficient and faster with
data lakes which is necessary for the rise in remote and hybrid working
environments. Data Lakes also offers an
open and secure platform as well.
The spotlight was on Data Engineering
As organizations aimed to increase their decision-making
process, the demand for data engineering along with data analysts grew. Similar to DevOps benefits for the
development teams, DataOps helped data engineers. Data Engineering teams developed storage and
transport strategies for hybrid and multi-cloud as well as edge
environments.
Data engineering plays a crucial role to refined the
processes of transforming, managing, and tracking the organization’s data for
better analysis leading to high demand for data engineers. The key sectors that leverage their services
are Banking and Insurance, Retail, eCommerce and Internet, Energy and
Industrialists, Pharma and Health, and Telecom among others.
Data quality challenges persisted
A significant number of organizations continued to
face the issues of data quality and validation aspects which are key to any
business performance. Data quality
challenges were seen in businesses throughout the year. Data quality challenges include inaccurate
data, outdated data, lack of standardization, duplicate and incomplete data,
and more. This was seen more in
organizations that used low-maturity DataOps practices where users were unsure
of the quality of data. This can be
addressed proactively and holistically with the appropriate strategies without
causing a high impact on the organization’s products and services. The need for
data quality systems and data regularization has given birth to companies that
provide solutions for these challenges. They
offer services in checking for data discrepancies and performing integrity
checks throughout the data Extract, Transform and Load (ETL) process.
The rise in the adoption of AI, ML, and Advanced
Analytics
Organizations today are acknowledging the power of
data and the value of data-driven decision-making than before. Traditional analytics methods do not have the
capabilities to analyze the massive amount of data that is generated every
day. An automated approach for data
analysis at scale is required. AI and ML
systems can help to spot patterns, detect anomalies in larger data sets, and
make accurate predictions through predictive analytics. With this, the
organization’s business processes can be improved and optimized which was not
possible earlier. AI and ML help
organizations to deliver customer support by leveraging intelligent chat bots
and providing personalized interactions, without the support of additional
customer service personnel. Along with
the implementation of a data lakes strategy, AI-enabled systems can gather and
analyze huge amounts of customer and user data which improves
decision-making. Data visualization is
also made possible with AI-enabled data analytics. Decision Intelligence provides several
options to deliver the advantages of eliminating biases, aiding data-driven
decisions, and also increasing the speed of decision-making processes. All organizations that leverage these
technologies that deliver real value, succeed in their digital marketing efforts.
In addition to the above, businesses also began to
pay more attention to data security, privacy, and governance aspects as
well. As the year 2022 saw more
organizations adopt data-driven models to their business processes, the data
analytics industry also witnessed huge growth and this trend is here to
stay. For all organizations who look at
taking their business to the next level, undoubtedly ‘data’ is providing the
opportunity.