Bridging the gap between finding insights and using them, with MLOps and ModelOps - Nilesh Satpute, Founder & CEO of Applied Cloud Computing
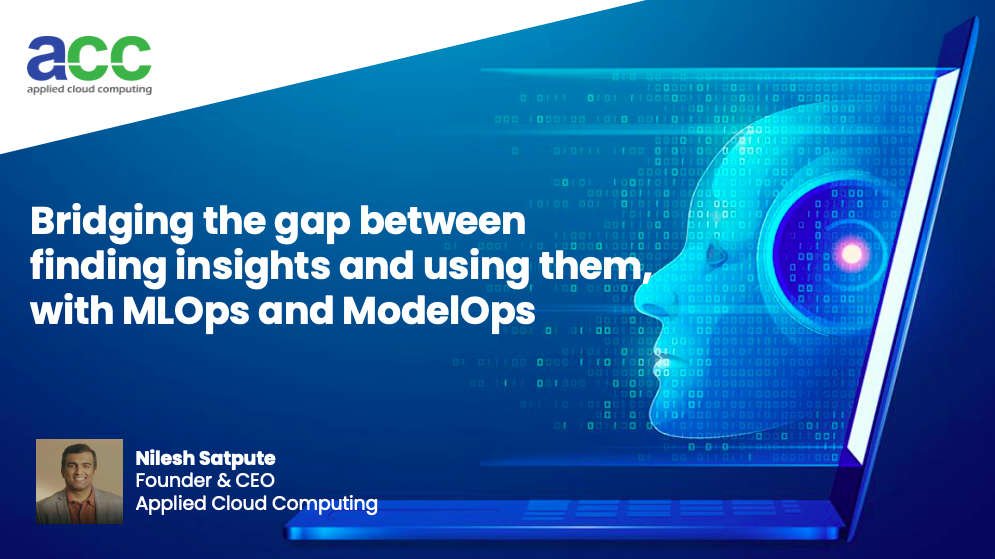
In the current VUCA world, business executives are increasingly seeking
technology solutions that allow them to make faster and better decisions, but
only if robust data back it. Many are leveraging Big Data and analytics to
support their project management initiatives, given that fears of a looming
recession make the C-suite cautious about every penny spent in the
organization.
At the same time, these professionals are willing to pull out all stops
when it comes to utilizing data to gain insights into processes that can
translate into business. This includes deep-diving into current internal
operations to understand their customer’s journey.
All this has increased spending globally on big data and business
analytics (BDA). According to IDC’s Worldwide Big Data and Analytics Spending
Guide 2021, the compound annual growth rate (CAGR) for worldwide BDA spending
over the 2021-2025 forecast period will be 12.8%. This figure is impressive,
considering the global economy recovered from the aftermath of the pandemic and
nosedived straight into a slowdown.
Straddling Two Boats
The accelerated digital transformation in the enterprise sector has also
seen data science gaining momentum. However, data scientists are now facing
varied challenges, key amongst which is resistance from decision-makers in
deployments and deficiency of data fluency in their companies.
In a recent SAS report, 42% of these professionals complained that
decision-makers often did not use their results, which negated the very purpose
of their role.
This is a surprise considering that many companies have invested heavily
in BDA, including collection, cleaning, storage and preparation of data.
According to the IDC report mentioned above, the US spent over $110 billion in
BDA in 2021, followed by Japan and China, where the BDA spending is forecast to
reach $12.4 billion and $11.9 billion, respectively.
Yet, data scientists opine that their efforts to sift through data to
discover perceptive patterns and build viable models are often wasted when
decision-makers do not leverage it to solve problems and take better decisions.
Deeplearning.ai reports that only 22% of companies using machine learning (ML)
have successfully deployed a model.
Fortunately, this chasm can be bridged by the two modern-day data
heroes—MLOps and ModelOps—words that every IT professional is happy to discuss
at length. So what do they represent, and how do enterprises gain by employing
it?
What Separates The
Two?
While MLOps and ModelOps might read and sound similar, they are very
distinctive. ModelOps is focused on utilizing enterprise-level data models,
which encompasses diverse groups in a company. While defining it, Gartner
claims that it focuses “primarily on the governance and life cycle management
of a wide range of operationalized artificial intelligence (AI) and decision
models, including machine learning, knowledge graphs, rules, optimization,
linguistic, linguistic and agent-based models”.
From an architecture perspective, ModelOps appears to be an extension of
MLOps and is often seen helping organize a software’s life cycle, including
creating a model, testing, deploying and monitoring it. This helps companies
better understand how to derive more from their AI investments.
And this is done in a manner that is simple. ModelOps provides decision
makers a dashboard, reporting, and other information to help them get a bird’s
eye view of any project’s progress. They can use this information to manage the
overall IT infrastructure as well as plan for future investments.
MLOps, on the other hand, is a subcategory application of DevOps
principles for ML models. Since it acts as the bridge between IT and software
development, MLOps aims to create recurrent and standardized processes for
using ML models. Data scientists use MLOps to collaborate and communicate
effectively while automating ML models.
Picking One Over The Other?
ModelOps is an enterprise exercise that helps business leaders improve
their decision-making by analyzing and understanding the model’s output and
deciphering whether and how it fits into their existing processes. For day-t0-day
decisions, they can create standardized workflows around how the model’s output
is managed, including end-users being able to question why a model made a
specific prediction.
Moreover, data scientists can put models into production faster and
actively monitor their performance using ML analytics. This is where ModelOps’
symbiotic relationship with MLOps comes into play. Using the duo helps data
scientists ensure that the model is viable, accurate and scalable.
Some MLOps solutions offer limited management capabilities, which
becomes apparent when a company starts scaling its AI and ML functions. It is
pertinent to work with service providers who can preset these ML models' risk,
regulatory and operational aspects.